Alright Sherlock, deduce.
Well, now that I have the majority of my results it is time for me to interpret what they mean!
So essentially I am stretching those lean detective muscles I’ve gained through watching so many crime shows in my 23 years. Deerstalker hat, magnifying glass and pipe at the ready; its time to get to work.
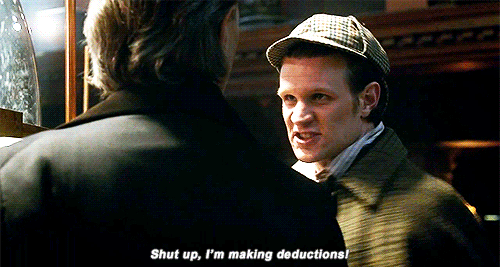
To date I have a formidable amount of tables, charts and maps generated. The long and short of it is, I was given a bunch of numbers and now I’m spitting back even more numbers but with some aesthetically pleasing pictures to accompany them.
There is my sensitivity analysis results to be discussed. Here the similarities between our two calculators are very clear. As well as the strengths and weakness I noticed while using them.
There is a small mountain of GHG emission estimations from both EX-ACT and SECTOR for a business as usual (BAU) 100% continuously flooded scenario and for a theoretical AWD scenario, where I set 40% of rice area to be converted to the revered mitigation practice, for interpretation. To accompany these estimations I have scatter-plots, bar-charts and maps to visualize the data in a much more concise manner.
An emissions factor or EF is a representative value that attempts to relate the quantity of a pollutant (methane in this case) released to the atmosphere with an activity associated with the release of that pollutant (i.e. rice cultivation).
For the emission estimations I just mentioned, I used a default EF set by the International Panel on Climate Change (IPCC) for all the provinces under analysis. So when I got a data set of the calculated EFs for each province of the MRD from IRRI I elected to recalculate the emission estimations again, to see how much the emission factor can affect the amount of GHG emissions. Short answer: Major effect.
The last piece of the puzzle I need now is the real world AWD area for me to make accurate estimations.
But for now I work with what I have! So far I have found that the two calculators selected at the outset of the project are very similar, they produce the close to the same results while requiring slightly different inputs to achieve the end result. The (linear) relationship is clear from my scatter-plots. So yes they are in fact related as I have stated before.
The maps I have produced are ideal for identifying the areas of highest emissions. The Mekong River Delta very clearly has higher emissions than the Red River Delta in this data set; likely due to the much larger areas of rice present in the area. The seasonal difference is also displayed in these maps; Autumn having the highest emissions while Winter shows the lowest. Again this is probably due to the areas planted differing each season.
A notable result when I input province and season specific EFs is that the winter season in the Mekong is in fact a mega emitter of GHG emissions. Completely contradicting the estimations I produced using the 1.3 default EF! This is mostly likely as a result of this season being the wet season; when rice paddys are flooded whether you like it or not.
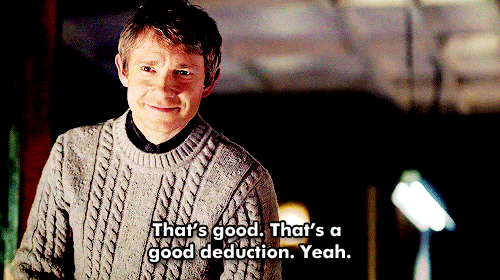
I would share my lovely figures and tables here but I’m afraid I will with-hold that until the thesis is fully written to give any solid conclusions. So keep an eye out for when I do get around to posting my results in their entirety!
Until then enjoy my mad ramblings as I pick them apart in my detective work!