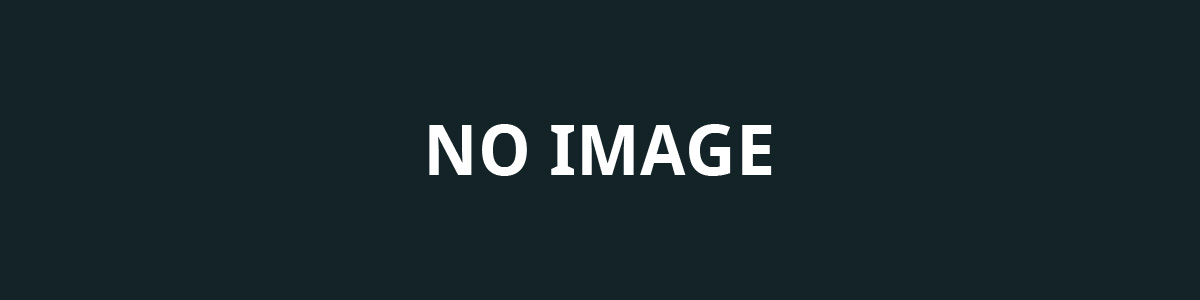
Crop modeling
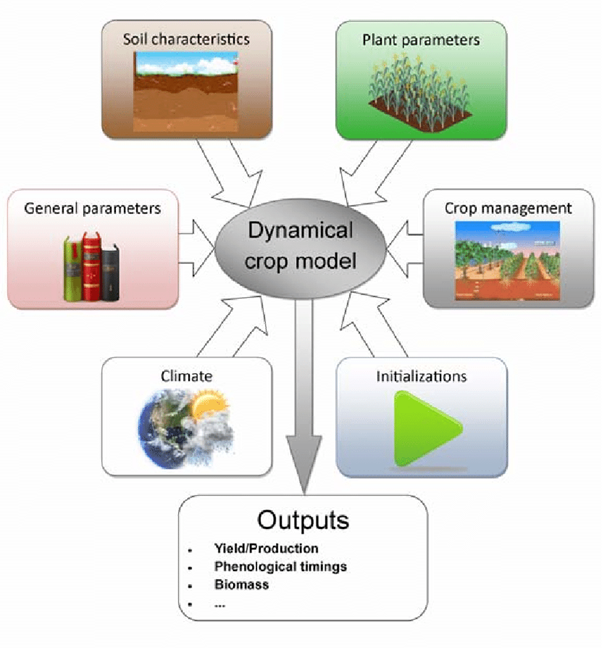
Crop models are computer programs that simulate the growth and development of crops by predicting the growth of individual components of a plant such as leaves, roots, stems, and grains. The modeling process uses equations or a series of equations to describe a system’s behavior (Oteng-Darko et al., 2013). The crop’s growth mechanisms are influenced by combining reactions and interactions between tissues and organs (Oteng-Darko et al., 2013). Crop models have been used in many research instances globally to measure climate impacts on crops (Zinyengere et al., 2015).
Why crop modeling?
Crop growth simulation models help evaluate the effects of climate change and agronomic management on the future crop production. As projections show a rise in the planet’s temperature with increased carbon dioxide and other greenhouse gas concentrations, global crop yields and productivity will be hampered (Nath et al., 2017). The effect on plants comes because plants’ biological processes of respiration, photosynthesis, plant development, reproduction, water use, and others are all affected by increased temperature and carbon dioxide concentrations. As a result, such effects will influence biomass partitioning and the plant’s overall growth and development. Therefore, crop modeling would aid scientists in guiding farmers’ choice of crop management decisions such as crop selection, cultivar selection, sowing regimes, and irrigation scheduling to reduce risks of crop failure and ensure resilient farming systems (Oteng-Darko et al., 2013).
As a research tool, model development and application can identify gaps in our knowledge, thus enabling more efficient and targeted research planning (Jones et al., 2003). The model can be used to effectively conduct research that would, in the end, save time and money spent in the field evaluating new cultivars and technologies. Therefore, crop modeling contributes to the speedy development of better sustainable agriculture practices that meet the world’s needs for food and other services (Oteng-Darko et al., 2013, Jones et al., 2003, Jones et al., 2016).
Model Parameterization
This concept involves calibration, evaluation, and validation of parameters in the model. Model calibration entails changing specific model parameters so that the data simulated by the error-free model matches the observed data. The model validation phase verifies that the calibrated model accurately depicts the real-world scenario. The technique entails comparing simulated performance and previous data from the calibration point (Jones et al., 2003). After one is confident that the models simulate the real world adequately, computer experiments are conducted hundreds or thousands of times for given environments to determine how to best manage or control the system (Jones et al., 2003).
Soybean Modelling Tools
Different crop models vary in their complexity and performance, thus their sensitivity to systematic variations in climatic change (Battisti, 2016, Battisti et al., 2018). These models are used for various purposes, including analyzing crop management practices in relation to meteorological conditions. Examples of models used for most plants are FAO – Agroecological Zone, AQUACROP, DSSAT CSM CROPGRO, APSIM, MONICA, PEGASUS, etc. These tools model future crop production as sole models or ensembles to give average projections.
The Decision Support System for Agrotechnology Transfer (DSSAT) Crop Simulation Model (CSM)
The DSSAT model is a software program that comprises dynamic crop growth simulation models (Jones et al., 2017). The International Benchmark Sites Network for Agrotechnology Transfer (IBSNAT) developed the tool and is one of the most widely used modeling tools (Jones et al., 2003). This network developed the tool after considering that the conventional agronomic research methods for generating data and publishing are insufficient to fulfill the growing research needs of modeling. Traditional agronomic studies are performed at specific times and locations, resulting in findings that are site and season-specific, time-consuming, costly, and under experimental trial and error (Jones et al., 2003). After ensuring that perhaps the models accurately replicate the real world, users can run computer experiments many times for specific conditions to decide the best way to operate or manipulate the device.
Dataset Required to do DSSAT Modelling
Various data is required in the process of running simulations as depicted in the figure above. however, the following are the primary datasets that are necessary to produce reasonable answers and to be easily collected in field situations(Jones et al., 2016):
- Data to Run the model
Weather data: Daily maximum/minimum temperature, precipitation, and solar radiation
Soil characteristics: Soil texture, bulk density, organic matter, nitrogen, and thickness for the soil horizon
Crop management data: Crop, cultivar/variety, planting date, row, plant spacing, irrigation dates and amounts, fertilizer and chemical applications, tillage operations, harvest.
- Data for Model Evaluation
Crop measurements: Yield and yield components (seed size and weights), phenology (flowering, physiological maturity dates).
For growth and partitioning: Biomass components (leaf, stem, seeds), Measurements of photosynthesis, canopy temperature
For Irrigation or fertilizer response: Soil measurements (moisture of different depths over time), soil nitrogen, Phosphorus, carbon.
References
BATTISTI, R. 2016. Calibration, uncertainties, and use of soybean crop simulation models for evaluating strategies to mitigate the effects of climate change in Southern Brazil. Universidade de São Paulo.
BATTISTI, R., SENTELHAS, P. & BOOTE, K. 2018. Sensitivity and requirement of improvements of four soybean crop simulation models for climate change studies in Southern Brazil. International journal of biometeorology, 62, 823-832.
JONES, J. W., ANTLE, J. M., BASSO, B., BOOTE, K. J., CONANT, R. T. & FOSTER, I. 2016. Brief history of agricultural systems modeling. Agricultural Systems, 155, 240-254.
JONES, J. W., ANTLE, J. M., BASSO, B., BOOTE, K. J., CONANT, R. T., FOSTER, I., GODFRAY, H. C. J., HERRERO, M., HOWITT, R. E. & JANSSEN, S. 2017. Brief history of agricultural systems modeling. Agricultural systems, 155, 240-254.
JONES, J. W., HOOGENBOOM, G., PORTER, C. H., BOOTE, K. J., BATCHELOR, W. D., HUNT, L., WILKENS, P. W., SINGH, U., GIJSMAN, A. J. & RITCHIE, J. T. 2003. The DSSAT cropping system model. European journal of agronomy, 18, 235-265.
NATH, A., KARUNAKAR, A., KUMAR, A., YADAV, A., CHAUDHARY, S. & SINGH, S. P. 2017. Evaluation of the CROPGRO-soybean model (DSSAT v 4.5) in the Akola region of Vidarbha, India. Ecology, Environment and Conservation, 23, 153-159.
OTENG-DARKO, P., YEBOAH, S., ADDY, S., AMPONSAH, S. & DANQUAH, E. O. 2013. Crop modeling: a tool for agricultural research–A. J Agric Res Dev, 2, 001-006.
ZINYENGERE, N., CRESPO, O., HACHIGONTA, S. & TADROSS, M. 2015. Crop model usefulness in drylands of southern Africa: an application of DSSAT. South African Journal of Plant and Soil, 32, 95-104.